Predicting Salinity in the Haringvliet Outer Delta
DOWNLOAD PROJECT SHEET
Calibrated and validated Machine Learning tool for predicting salinity levels, Artificial timeseries of 90 years of salinity levels constructed with the ML tool

Deltares

Haringvliet

2024
Using Machine Learning for operational and long-term predictions of salinity
As part of the research program “Onderzoeksprogramma Verzilting van Lerend Implementeren Kierbesluit Haringvlietsluizen”, fish migration into the Haringvliet should be stimulated within feasible boundary conditions of inland salinity penetration. Therefore, simulations and field studies are conducted to investigate salt dispersion in the Haringvliet estuary.
A critical parameter for this research is the salinity in the Haringvliet Outer Delta (‘Voordelta’). The Outer Delta is located on the seaside of the Haringvliet barrier, which separates the Haringvliet from the sea. Despite the abundance of measurement data, predicting this salinity level remains challenging. Current methods like detailed 3D modelling are highly resource-intensive and costly.
The extensive dataset on salinity levels in the Outer Delta offers the potential for leveraging Machine Learning (ML) models for predictive purposes, potentially leading to cost reductions. This study investigates and applies feasible ML models to address this challenge. With minimal investment, the aim is to demonstrate the potential of applying ML models to predict complex processes, such as salinity dynamics in estuarine systems.
We selected a Random Forest Regression model and Extreme Gradient Boosting model (XGBoost) as suitable models for the application of the tool. The five-year salinity dataset was divided into a training set (48 months), a calibration set (2.5 months), and a test set (3.5 months). Input parameters were selected based on an exploratory data analysis and feature selection using Recursive Feature Elimination with Cross-Validation (RFECV).
Both models are capable of predicting the salinity levels with a surprising accuracy, with a lowest Mean Absolute Error of 390 mg/L for the XGBoost model. Model performance was found to depend primarily on the discharge rate of the Haringvliet sluices. The model exhibited the highest accuracy at low discharges, but could occasionally underestimate salinity peaks at moderate discharges.
Yannick Steenman
Project leader
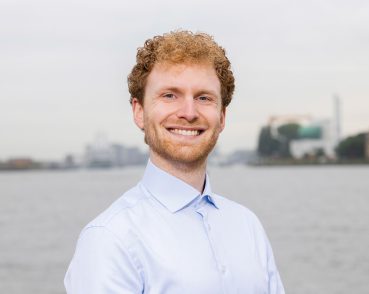
More information about Svašek or our expertises?
Please contact us or make an appointment.
CONTACT US